4卡3090平台的带宽测试
写的有点乱
在6032机器上进行一些PCIe传输的实验
1.机器配置
- GPU0与GPU1在同一个NUMA节点
- GPU2与GPU3分别在另外两个NUMA节点
首先写一个简单的验证卡间PA(peer access)功能的CUDA程序:
#include <iostream>
#include <cuda_runtime.h>
int main() {
int deviceCount = 0;
cudaGetDeviceCount(&deviceCount);
if (deviceCount < 2) {
std::cout << "P2P requires at least two GPUs.\n";
return 0;
}
for (int i = 0; i < deviceCount; ++i) {
for (int j = 0; j < deviceCount; ++j) {
if (i != j) {
int p2pCapable = 0;
cudaDeviceCanAccessPeer(&p2pCapable, i, j);
if (p2pCapable) {
std::cout << "Device " << i << " can access device " << j << " memory directly.\n";
} else {
std::cout << "Device " << i << " cannot access device " << j << " memory directly.\n";
}
}
}
}
return 0;
}
在0号和1号卡之间和2号和3号卡之间安装NVLink时测试一下PA结果如下:
$ ./test
Device 0 can access device 1 memory directly.
Device 0 cannot access device 2 memory directly.
Device 0 cannot access device 3 memory directly.
Device 1 can access device 0 memory directly.
Device 1 cannot access device 2 memory directly.
Device 1 cannot access device 3 memory directly.
Device 2 cannot access device 0 memory directly.
Device 2 cannot access device 1 memory directly.
Device 2 can access device 3 memory directly.
Device 3 cannot access device 0 memory directly.
Device 3 cannot access device 1 memory directly.
Device 3 can access device 2 memory directly.
可见RTX 3090实际上是不支持基于PCIe的PA传输的,这个我还没想好怎么解决,为了暂时排除NVLink对我的误导(可能在程序上有疏忽没有禁用NVLink),我先把桥接器拆掉了,等需要的时候再使用,此时执行:
$ nvidia-smi
GPU | Name | Persistence-M | Bus-Id | Disp.A | Volatile Uncorr. ECC | Fan | Temp | Perf | Pwr:Usage/Cap | Memory-Usage | GPU-Util | Compute M. |
---|---|---|---|---|---|---|---|---|---|---|---|---|
0 | NVIDIA GeForce RTX 3090 | Off | 00000000:41:00.0 | Off | N/A | 44% | 55C | P0 | 131W / 350W | 0MiB / 24576MiB | 0% | Default |
1 | NVIDIA GeForce RTX 3090 | Off | 00000000:42:00.0 | Off | N/A | 30% | 42C | P0 | 103W / 350W | 0MiB / 24576MiB | 0% | Default |
2 | NVIDIA GeForce RTX 3090 | Off | 00000000:81:00.0 | Off | N/A | 37% | 49C | P0 | 116W / 350W | 0MiB / 24576MiB | 0% | Default |
3 | NVIDIA GeForce RTX 3090 | Off | 00000000:C1:00.0 | Off | N/A | 39% | 58C | P0 | 125W / 350W | 0MiB / 24576MiB | 0% | Default |
$ nvidia-smi topo -m
GPU0 | GPU1 | GPU2 | GPU3 | CPU Affinity | NUMA Affinity | |
---|---|---|---|---|---|---|
GPU0 | X | PHB | SYS | SYS | 32-47,96-111 | 2 |
GPU1 | PHB | X | SYS | SYS | 32-47,96-111 | 2 |
GPU2 | SYS | SYS | X | SYS | 16-31,80-95 | 1 |
GPU3 | SYS | SYS | SYS | X | 0-15,64-79 | 0 |
$ nvidia-smi -q | grep -A 10 "PCI"
GPU ID | PCIe 总线 ID | PCIe 代数 | 链路宽度 | 当前链路宽度 | 温度 |
---|---|---|---|---|---|
GPU 0 | 41 | 4.0 | 16x | 16x | 51°C |
GPU 1 | 42 | 3.0 | 16x | 16x | 40°C |
GPU 2 | 81 | 4.0 | 16x | 16x | 50°C |
GPU 3 | C1 | 4.0 | 16x | 16x | 58°C |
此时再执行上面的PA测试程序则全部不可访问:
Device 0 cannot access device 1 memory directly.
Device 0 cannot access device 2 memory directly.
Device 0 cannot access device 3 memory directly.
Device 1 cannot access device 0 memory directly.
Device 1 cannot access device 2 memory directly.
Device 1 cannot access device 3 memory directly.
Device 2 cannot access device 0 memory directly.
Device 2 cannot access device 1 memory directly.
Device 2 cannot access device 3 memory directly.
Device 3 cannot access device 0 memory directly.
Device 3 cannot access device 1 memory directly.
Device 3 cannot access device 2 memory directly.
2.带宽测试的异常
现在以无NVLink的模式进行带宽测试,使用CUDA API cudaMemcpy(Async)
的 cudaMemcpyDeviceToDevice
进行数据的Device-to-Device
传输,完整程序如下:
#include <iostream>
#include <vector>
#include <cuda_runtime.h>
#define checkCudaErrors(val) check((val), #val, __FILE__, __LINE__)
void check(cudaError_t result, const char *func, const char *file, int line) {
if (result != cudaSuccess) {
std::cerr << "CUDA error at " << file << ":" << line << " code=" << result
<< " \"" << func << "\" " << cudaGetErrorString(result) << std::endl;
exit(EXIT_FAILURE);
}
}
int main() {
int deviceCount;
checkCudaErrors(cudaGetDeviceCount(&deviceCount));
if (deviceCount < 4) {
std::cerr << "This program requires at least four CUDA-capable devices.\n";
return EXIT_FAILURE;
}
// 定义需要传输的数据大小 (1 GB) 和设备对
size_t dataSize = 1 << 28; // 1 GB 数据(268,435,456 个 float)
std::vector<std::pair<int, int>> devicePairs;
// 创建所有 GPU 对之间的组合
for (int i = 0; i < deviceCount; i++) {
for (int j = 0; j < deviceCount; j++) {
if (i != j) {
devicePairs.push_back({i, j});
}
}
}
// 初始化每个传输的资源
std::vector<float*> d_srcs(devicePairs.size());
std::vector<float*> d_dsts(devicePairs.size());
std::vector<cudaStream_t> streams(devicePairs.size());
std::vector<cudaEvent_t> startEvents(devicePairs.size());
std::vector<cudaEvent_t> stopEvents(devicePairs.size());
std::vector<float> totalMilliseconds(devicePairs.size(), 0);
// 为每对设备分配内存和流
for (size_t i = 0; i < devicePairs.size(); i++) {
int fromDevice = devicePairs[i].first;
int toDevice = devicePairs[i].second;
// 在源设备上分配内存并创建流
checkCudaErrors(cudaSetDevice(fromDevice));
checkCudaErrors(cudaMalloc(&d_srcs[i], dataSize * sizeof(float)));
checkCudaErrors(cudaStreamCreate(&streams[i]));
// 在目标设备上分配内存
checkCudaErrors(cudaSetDevice(toDevice));
checkCudaErrors(cudaMalloc(&d_dsts[i], dataSize * sizeof(float)));
// 创建事件用于测量时间
checkCudaErrors(cudaSetDevice(fromDevice));
checkCudaErrors(cudaEventCreate(&startEvents[i]));
checkCudaErrors(cudaEventCreate(&stopEvents[i]));
}
// 执行设备到设备的异步内存传输
for (size_t i = 0; i < devicePairs.size(); i++) {
int fromDevice = devicePairs[i].first;
int toDevice = devicePairs[i].second;
// 设置源设备并记录传输开始时间
checkCudaErrors(cudaSetDevice(fromDevice));
checkCudaErrors(cudaEventRecord(startEvents[i], streams[i]));
// 异步复制数据(设备到设备)
checkCudaErrors(cudaMemcpyAsync(d_dsts[i], d_srcs[i], dataSize * sizeof(float),
cudaMemcpyDeviceToDevice, streams[i]));
// 记录传输结束时间
checkCudaErrors(cudaEventRecord(stopEvents[i], streams[i]));
}
// 同步流并计算每对设备的传输时间和带宽
for (size_t i = 0; i < devicePairs.size(); i++) {
int fromDevice = devicePairs[i].first;
int toDevice = devicePairs[i].second;
// 设置源设备并同步流
checkCudaErrors(cudaSetDevice(fromDevice));
checkCudaErrors(cudaStreamSynchronize(streams[i]));
// 计算传输时间
float milliseconds = 0;
checkCudaErrors(cudaEventElapsedTime(&milliseconds, startEvents[i], stopEvents[i]));
totalMilliseconds[i] = milliseconds;
// 计算带宽 (GB/s)
float bandwidth = (dataSize * sizeof(float) / (1 << 30)) / (milliseconds / 1000.0f);
// 打印传输时间和带宽
std::cout << "Transfer from Device " << fromDevice << " to Device " << toDevice
<< ": " << milliseconds << " ms, Bandwidth: " << bandwidth << " GB/s\n";
}
// 清理资源
for (size_t i = 0; i < devicePairs.size(); i++) {
checkCudaErrors(cudaFree(d_srcs[i]));
checkCudaErrors(cudaFree(d_dsts[i]));
checkCudaErrors(cudaStreamDestroy(streams[i]));
checkCudaErrors(cudaEventDestroy(startEvents[i]));
checkCudaErrors(cudaEventDestroy(stopEvents[i]));
}
return 0;
}
测试的结果却有些奇怪:
可见这个带宽远远没有达到Gen3 x16(16GB/s)或Gen4 x16(32GB/s)的单向传输带宽,我怀疑是我的程序写的有问题(不是很熟悉CUDA编程),因此下载了官方的链路测试工具 NCCL-tests并进行一些通信原语的测试:
- All-Reduce
- All-Gather
- BroadCast
- Reduce-Scatter
可见带宽依然表现不是很正常,但NCCL的集合通信测试似乎是包含环拓扑与树拓扑,带宽降低也许是被GPU1的Gen3链路限制了,因此我在测试命令前添加了CUDA_VISIBLE_DEVICES=2,3
环境变量来指定Gen4链路的GPU2和GPU3来进行新的测试,但结果All-Reduce与Reduce-Scatter有1倍左右的性能提升,BroadCast有70%左右的性能提升,All-Gather几乎没有变化,这个现象说明Gen4确实比环拓扑中存在Gen3的链路有近乎1倍的提升,这样的结果与在网上看的其他博客就差不多了。
我发现每次执行CUDA程序都要等待1、2秒才开始,这是因为GPU没有开启Persistent Mode,执行
sudo nvidia-smi -pm 1
开启后程序就能“秒执行”了这并不会对程序的性能有任何影响(在我一些简单的测试后)
3.数据传输带宽问题分析
-
CUDA数据传输的优化参考:How to Optimize Data Transfers in CUDA C/C++
-
CUDA数据传输的重叠参考:How to Overlap Data Transfers in CUDA C/C++
首先需要排除我代码上的错误,因此我去看了手撸一下GPU D2D实现(PCIe版)的测试源码,并用这份源码进行了带宽的测试:
可见结果还是不理想,值得注意的是从这个结果可以看出跨NUMA节点确实会导致带宽下降–[1-3]/[1-0]的两组结果表示产生了**8.75%**的带宽下降,这个结果让我想到了之前看到的一篇文章ASPLOS'24-TCCL: Discovering Better Communication Paths for PCIe GPU Clusters中的一个结果:
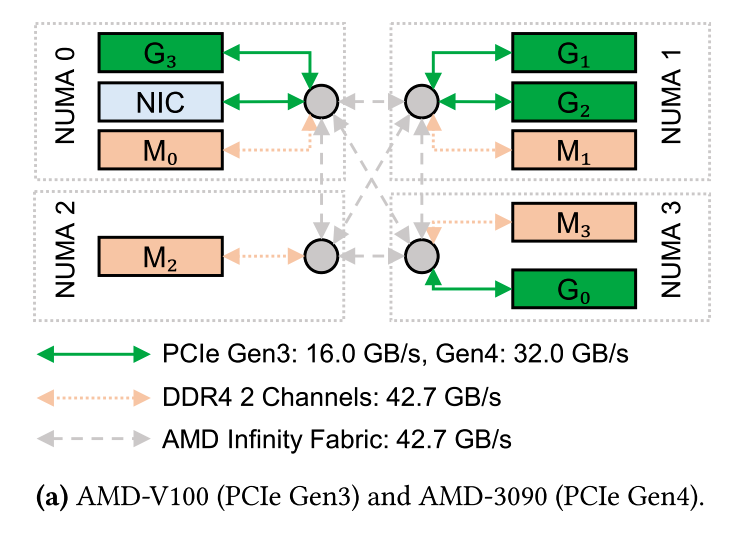
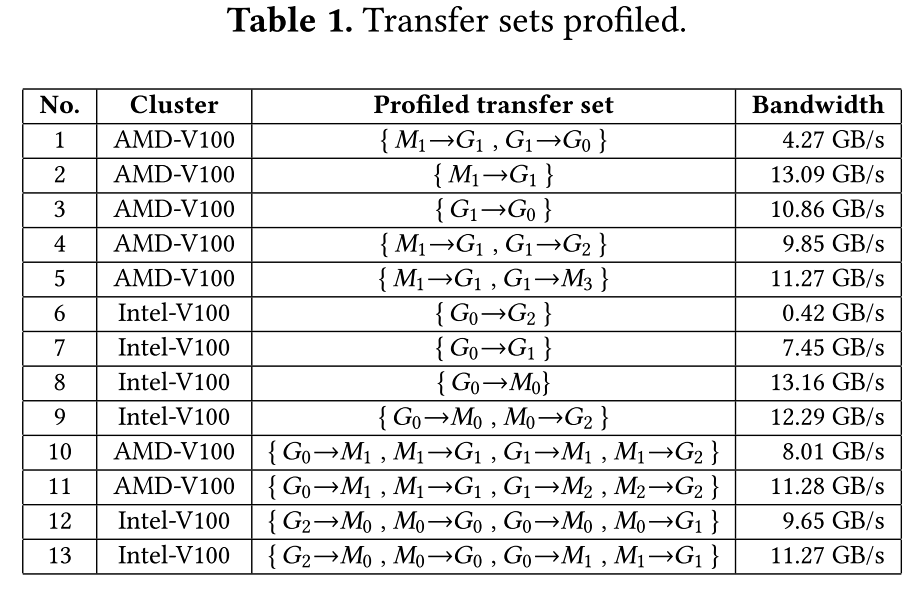
注意看结果4与结果5的**12.5%**带宽下降,实验条件的不完全一致或许是差值的由来,但趋势却能表现出来。
通过阅读How to Optimize Data Transfers in CUDA C/C++我尝试写了一个新的带宽测试程序,分别测试可分页内存和页锁定内存的数据传输带宽,完整代码如下:
#include <cuda_runtime.h>
#include <iostream>
#include <nvtx3/nvToolsExt.h> // NVTX 库头文件
void profileCopies(float* h_a, float* h_b, float* d, unsigned int n, const char* desc) {
std::cout << desc << " transfers\n";
unsigned int bytes = n * sizeof(float);
cudaEvent_t startEvent, stopEvent;
cudaEventCreate(&startEvent);
cudaEventCreate(&stopEvent);
// NVTX Range for Host to Device transfer
std::string rangeName = std::string(desc) + " Host to Device Transfer";
nvtxRangePush(rangeName.c_str());
// Host to Device transfer
cudaEventRecord(startEvent, 0);
cudaMemcpy(d, h_a, bytes, cudaMemcpyHostToDevice);
cudaEventRecord(stopEvent, 0);
cudaEventSynchronize(stopEvent);
float time;
cudaEventElapsedTime(&time, startEvent, stopEvent);
std::cout << " Host to Device bandwidth (GB/s): " << bytes * 1e-6 / time << "\n";
// Close NVTX Range for Host to Device transfer
nvtxRangePop();
// NVTX Range for Device to Host transfer
rangeName = std::string(desc) + " Device to Host Transfer";
nvtxRangePush(rangeName.c_str());
// Device to Host transfer
cudaEventRecord(startEvent, 0);
cudaMemcpy(h_b, d, bytes, cudaMemcpyDeviceToHost);
cudaEventRecord(stopEvent, 0);
cudaEventSynchronize(stopEvent);
cudaEventElapsedTime(&time, startEvent, stopEvent);
std::cout << " Device to Host bandwidth (GB/s): " << bytes * 1e-6 / time << "\n";
// Close NVTX Range for Device to Host transfer
nvtxRangePop();
cudaEventDestroy(startEvent);
cudaEventDestroy(stopEvent);
}
int main() {
// 设置设备为 GPU 3
cudaSetDevice(3); // 指定 GPU 3
const unsigned int nElements = 4 * 1024 * 1024;
const unsigned int bytes = nElements * sizeof(float);
// Start NVTX Range for main function
nvtxRangePush("Main Function");
// NVTX Range for Memory Allocation
nvtxRangePush("Memory Allocation");
float *h_aPageable = (float*)malloc(bytes);
float *h_bPageable = (float*)malloc(bytes);
float *h_aPinned, *h_bPinned;
float *d_a;
// Allocate pageable and pinned host memory
cudaMallocHost(&h_aPinned, bytes);
cudaMallocHost(&h_bPinned, bytes);
cudaMalloc(&d_a, bytes);
nvtxRangePop(); // End NVTX Range for Memory Allocation
// NVTX Range for Memory Initialization
nvtxRangePush("Memory Initialization");
// Initialize host memory
for (int i = 0; i < nElements; ++i) h_aPageable[i] = i;
memcpy(h_aPinned, h_aPageable, bytes);
nvtxRangePop(); // End NVTX Range for Memory Initialization
// Pageable transfer with NVTX Range
nvtxRangePush("Pageable Memory Transfer");
profileCopies(h_aPageable, h_bPageable, d_a, nElements, "Pageable");
nvtxRangePop(); // End NVTX Range for Pageable Memory Transfer
// Pinned transfer with NVTX Range
nvtxRangePush("Pinned Memory Transfer");
profileCopies(h_aPinned, h_bPinned, d_a, nElements, "Pinned");
nvtxRangePop(); // End NVTX Range for Pinned Memory Transfer
// NVTX Range for Cleanup
nvtxRangePush("Cleanup");
// Cleanup
cudaFree(d_a);
cudaFreeHost(h_aPinned);
cudaFreeHost(h_bPinned);
free(h_aPageable);
free(h_bPageable);
nvtxRangePop(); // End NVTX Range for Cleanup
nvtxRangePop(); // End NVTX Range for Main Function
return 0;
}
测试结果如下:
$ nsys profile --trace=cuda,osrt,nvtx -o memtrans_profile ./memtrans
这里的运行命令是生成Nsight system中可分析的
.nsys-rep
文件,以帮助我定位问题
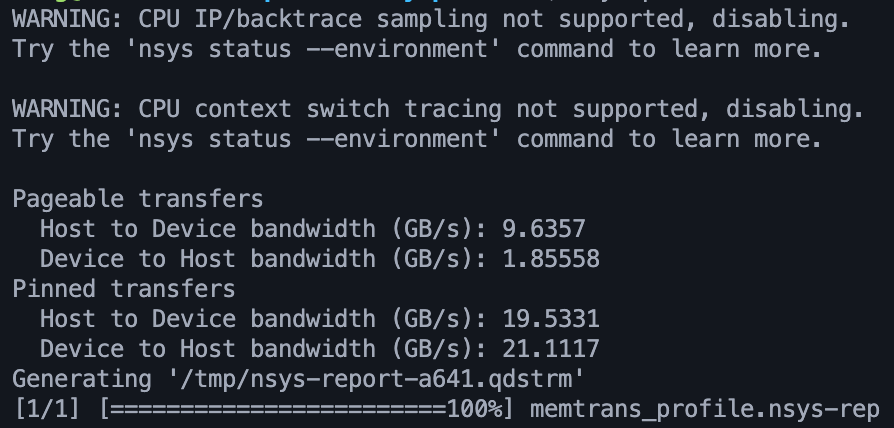
可见如果是页锁定内存,则带宽表现是正常的(Gen4 x16全速的60%左右),而可分页内存的带宽表现则差很多,这是正常的,但可分页内存的Device to Host Bandwidth
表现非常差。
为了避免所有程序上的干扰,我使用NV提供的CUDA例程进行BW测试,结果如下:
设备 | 节点 | 描述 | 访问方式 | 内存类型 | 带宽 (GB/s) |
---|---|---|---|---|---|
GPU0 | NODE2 | Gen4 x16 | DtoH | Pageable | 4.6 |
Pinned | 19.3 | ||||
HtoD | Pageable | 11.7 | |||
Pinned | 14.5 | ||||
GPU1 | NODE2 | Gen3 x16 | DtoH | Pageable | 4.3 |
Pinned | 12.1 | ||||
HtoD | Pageable | 11.4 | |||
Pinned | 11 | ||||
GPU3 | NODE0 | Gen4 x16 | DtoH | Pageable | 4.7 |
Pinned | 19.5 | ||||
HtoD | Pageable | 11.8 | |||
Pinned | 14.7 |
我自己写的D2H的带宽表现过于差,因此我学习了How to Optimize Data Transfers in CUDA C/C++中的代码写法,在数据传输前使用memset(h_bPageable, 0, bytes)
对host上分配的内存进行初始化再进行传输,代码如下:
#include <stdio.h>
#include <cuda_runtime.h>
int main() {
const unsigned int N = 1048576; // 定义常量 N,表示数组的元素数量(1,048,576)
const unsigned int bytes = N * sizeof(int); // 计算数组占用的字节数,4,194,304 字节
int *h_a = (int*)malloc(bytes); // 在主机上分配内存
int *d_a; // 定义设备指针
cudaMalloc((int**)&d_a, bytes); // 在设备上分配内存
memset(h_a, 0, bytes); // 初始化主机数组为0
cudaEvent_t start, stop;
float elapsedTime;
// 创建事件
cudaEventCreate(&start);
cudaEventCreate(&stop);
// 测量 Host to Device 的带宽
cudaEventRecord(start, 0); // 记录开始时间
cudaMemcpy(d_a, h_a, bytes, cudaMemcpyHostToDevice); // 从主机到设备的数据复制
cudaEventRecord(stop, 0); // 记录结束时间
cudaEventSynchronize(stop); // 等待事件完成
cudaEventElapsedTime(&elapsedTime, start, stop); // 计算经过的时间(毫秒)
float h2d_bandwidth = (bytes / (1024.0 * 1024.0 * 1024.0)) / (elapsedTime / 1000.0); // 计算带宽 GB/s
printf("Host to Device Bandwidth: %.2f GB/s\n", h2d_bandwidth);
// 测量 Device to Host 的带宽
cudaEventRecord(start, 0); // 记录开始时间
cudaMemcpy(h_a, d_a, bytes, cudaMemcpyDeviceToHost); // 从设备到主机的数据复制
cudaEventRecord(stop, 0); // 记录结束时间
cudaEventSynchronize(stop); // 等待事件完成
cudaEventElapsedTime(&elapsedTime, start, stop); // 计算经过的时间(毫秒)
float d2h_bandwidth = (bytes / (1024.0 * 1024.0 * 1024.0)) / (elapsedTime / 1000.0); // 计算带宽 GB/s
printf("Device to Host Bandwidth: %.2f GB/s\n", d2h_bandwidth);
// 清理
cudaEventDestroy(start);
cudaEventDestroy(stop);
cudaFree(d_a);
free(h_a);
return 0;
}
这段程序测出的带宽表现比较正常了:
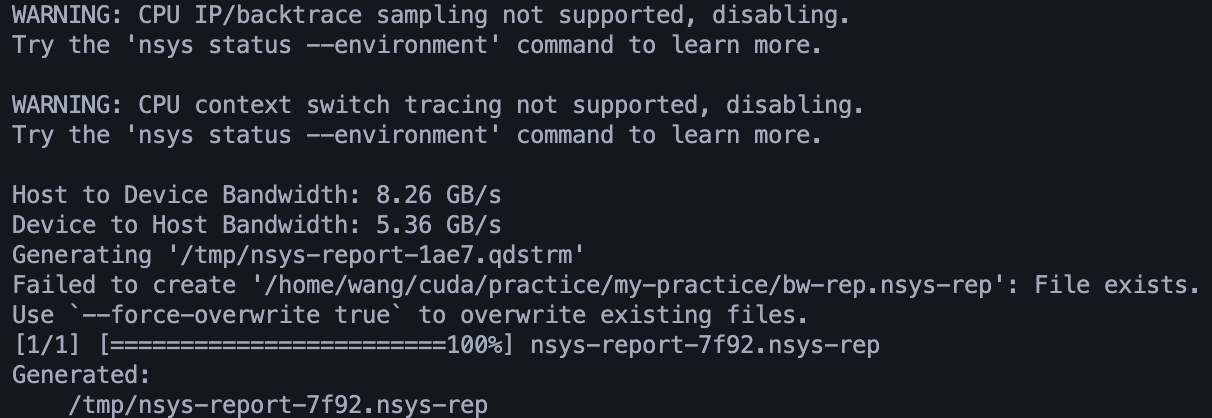
将生成的nsys-rep文件导入到Nsight system看一下:
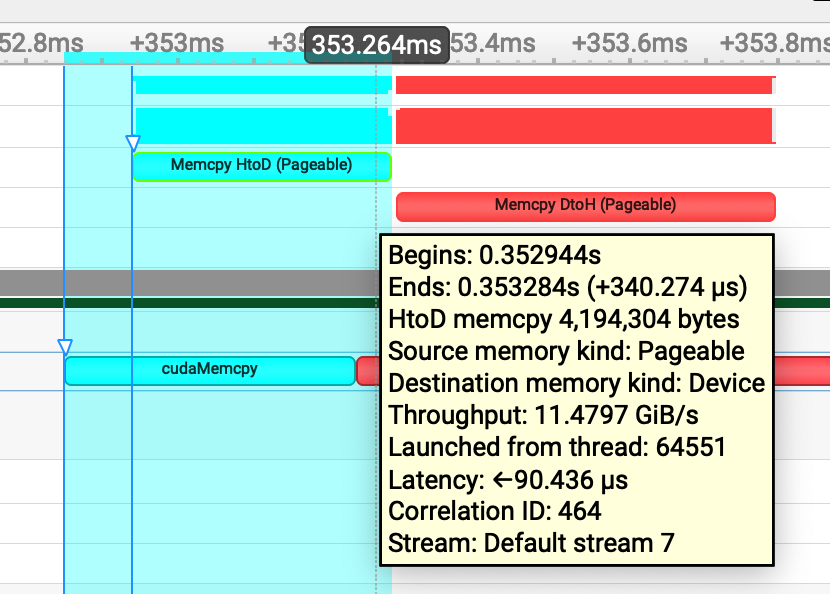
可见Memcpy HtoD是在cudaMemcpy函数调用之后若干毫秒后开始的,因此程序测出的带宽比实际带宽略低。
现在pageable和pinned的问题基本搞清楚了,但是手撸一下GPU D2D实现(PCIe版)的测试源码在我的平台上表现的还是不如原文中4090系统的第一个简单实验的带宽高,反而是后续答主自己做的CPU主存buffer中转带宽表现与我的3090差不多,在答主的源码中使用的是cudaMemcpyAsync
这个函数进行PA传输,我看了下Nsight system这个函数的流,确实就是先HtoD再DtoH,但在我的Nsight中只看带宽感觉像是使用的pageable内存,为什么答主的4090使用cudaMemcpyAsync
进行直接传输的带宽这么高,而答主自己进行buffer优化的带宽又下降了,我怀疑是cudaMemcpyAsync
在4090中自动进行了PA传输,答主认为4090是不支持PA的,但实际上4090支持基于PCIe的PA,3090是一定不支持的,必须通过NVLink。
在基本掌握我这台GPU试验台的数据传输性能,并熟悉CUDA在数据传输方面的代码编写后,我不准备深入优化这一部分了(实际上NCCL早就把这些简单的东西做完了)。
但3090如何进行基于PCIe的PA(通过switch实现)以及NV的GPU核心如何访问L2 Cache的,还需进一步探索。
看到了一个与我具有类似困惑的Issues我决定先在BIOS里将IOMMU关闭再试试。
IOMMU关闭后运行
NCCL_P2P_DISABLE=1 CUDA_VISIBLE_DEVICES=2,3 ./build/broadcast_perf -b 8 -e 128M -f 2 -g 2
的结果比之前糟糕,这是为什么呢,按理说开启IOMMU只会让HOST卡性能降低,我再把IOMMU打开试试。
把IOMMU打开后还是没有什么效果,不知道是硬件哪里出了问题,不过性能的下降并不多,暂不深究了。